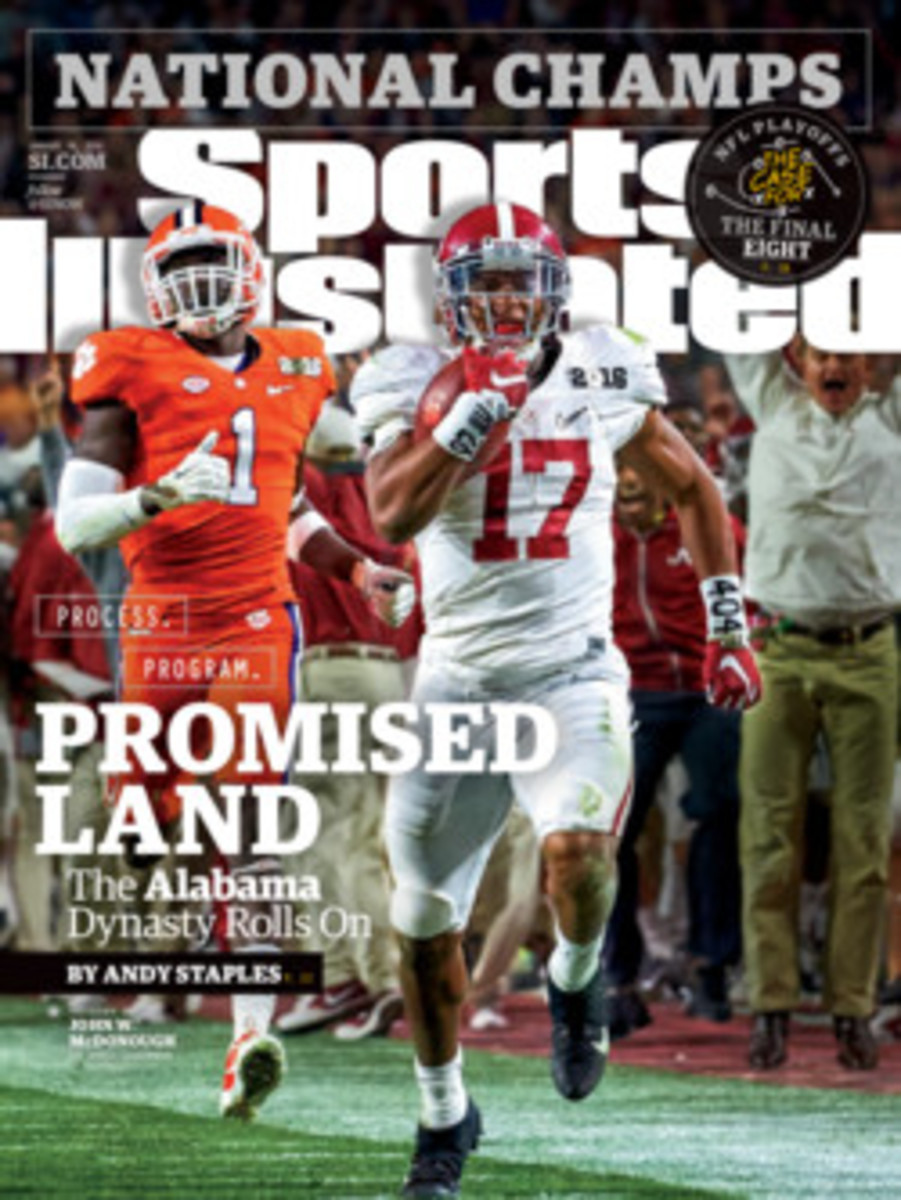
OK COMPUTER
As part of an ongoing series imagining the next 50 years of football, SI and Wired look at how computers will—and won't—change the game
In 1996, IBM's Deep Blue became the first supercomputer to defeat a chess grandmaster, Garry Kasparov, in a game. A year later Deep Blue edged Kasparov 3½--2½ in a full match. Why should you, a football fan, care? Because, as the late linebacker Junior Seau once said, "football is a chess game."
Deep Blue defeated Kasparov by brute force, scanning through 200 million moves per second. And modern smartphones make even Deep Blue look painfully slow: A Samsung Galaxy S5, for example, can perform 140 billion floating-point operations per second, more than 10 times the speed of IBM's old machine. Moore's Law predicts that computational power doubles roughly every two years, so by Super Bowl 100, in 2066, computers should be several million times faster than today. Imagine a robot Bill Belichick flicking through a digital playbook of trillions of moves during the 40-second gap between plays.
The application of artificial intelligence to play-calling is already upon us. Before this season SI approached a big-data analysis company, Splunk, and asked whether it could forecast the next play. Nate McKervey, Splunk's director of technical marketing, plugged 15 years' worth of NFL data into his company's platform. On Nov. 8, with the Falcons at their own 46-yard line with 5:21 to go in the third quarter, McKervey's friend asked him to make a prediction. McKervey glanced at his phone. It showed that Atlanta would line up in shotgun formation, and quarterback Matt Ryan would pass short left. Although the throw was incomplete, everything matched perfectly.
Football, of course, is more complicated than pass-versus-run. The number of possible scenarios on a field far outnumber the options on a chess board: Players aren't confined to squares, and the pawns along the line of scrimmage or the rooks split wide come in a variety of sizes.
The two AI research areas with the most potential relevance for football are machine learning and game theory. Machine learning, says Yoav Shoham, a professor emeritus of computer science at Stanford and a principal scientist at Google, is "Moneyball on steroids." Given a large set of data, a clever computer can search for patterns and learn from past actions and outcomes. If everyone always punts on fourth-and-long, the computer will always punt on fourth-and-long.
The power of this approach lies in the size of the data set—the more information available, the smarter the computer. But data gaps can be a problem. And teams keep secrets from one another. Game theory, in contrast, works best when there are knowledge gaps. According to Matthew O. Jackson, a professor of economics at Stanford who teaches an online game theory course with Shoham, last year's Super Bowl was a perfect example. "If you're the Seahawks, you can't just always give it to [Marshawn] Lynch because the other team can just key off that," Jackson says. "And if you're the Patriots, you can't just assume [Seattle is] going to give it to Lynch." Sometimes unexpectedly playing the weaker of two options is better—such as going for it on fourth-and-long.
But while machine learning might fine-tune scouting reports, and game theory might offer situational options, both are ultimately just tools for a coach to employ. And in 2066, that coach will still be human. Probably.
SI.COM
Got more pigskin-pointed questions for our crystal ball? You can further explore the future of football with our Super Bowl 100 series, presented by Gatorade and Microsoft Surface, at SI.com/sb100 and Wired.com/sb100
PHOTO
JIM ROGASH/GETTY IMAGES
THE B-1000 Though computers are getting exponentially more powerful, they won't replace coaches like Belichick anytime soon.